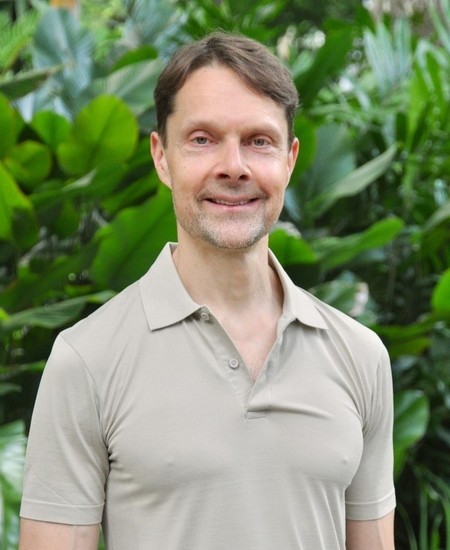
This work package will deploy technology to assist in manpower training in ways that are not currently adopted in the aviation industry. Specifically, the projects will use eye tracking technology to quantify and personalize training programs for pilots, and use augmented reality (AR) and virtual reality (VR) technology to complement existing training programs and to provide SIA with greater learning flexibility.
This project aims to create a data- and technology-driven and competency-based training framework for pilot and cabin crew training.
The team will use eye tracking technology to develop a data-driven and competency-based training framework which will provide an objective approach to training, potentially reduce the number of required hours in flight simulators, and reduce training cost.
This technology aims to objectively measure performance, provide personalized training and reduce cost by giving SIA ability to transport and replicate this training beyond a centralized location.
In order to provide a high-quality flight experience, it is necessary to plan the optimal schedule for the crew and to provide immediate feedback post-flight. Current scheduling and roster solutions use a deterministic and blanket strategy whereby all crew are treated equal. Existing algorithms optimize based on constraints without the consideration of crew preferences and performance. As a result, the team propose an intelligent scheduling system which takes into account individual-level factors including preferences and performance.
The use of AR and VR will help to complement existing training programs for new cadets as well as routine refresher courses for existing flight and cabin crew by providing greater flexibility in where they can train, and the types of scenarios and situations which can be simulated beyond existing methods.
In this project, proven causal inference techniques will be used to identify environmental factors and people attributes that contribute to positive training and flight outcomes. Combining the insights of our models with the existing rostering data from Jeppesen and call center enquiries, an optimal scheduling algorithms will be designed to simultaneously satisfy the preferences of the crew and achieve optimal flight outcomes.
Jussi Keppo, Ville Satopää
Problem Statement: This article analyzes multiple agents who forecast an underlying dynamic state based on streams of (partially overlapping) information. Each agent minimizes a convex combination of their forecasting error and deviation from the other agents' forecasts. As a result, the agents exhibit herding behavior - a bias that has been well-recognized in the economics and psychology literature. Our first contribution is a general framework for analyzing agents' forecasts under different levels of herding. The underlying state dynamics can be non-linear with seasonality, trends, shocks, or other time-series components....
This research is supported by National Research Foundation, Singapore and A*STAR, under its RIE2020 Industry Alignment Fund – Industry Collaboration Projects (IAF-ICP) grant call (Grant No. I2001E0059)
Jia-Wei Liu, Yan-Pei Cao, Weijia Mao, Wenqiao Zhang, David Junhao Zhang, Jussi Keppo, Ying Shan, Xiaohu Qie, Mike Zheng Shou
Problem Statement: Modeling dynamic scenes is important for many applications such as virtual reality
and telepresence. Despite achieving unprecedented fidelity for novel view synthesis
in dynamic scenes, existing methods based on Neural Radiance Fields (NeRF)
suffer from slow convergence (i.e., model training time measured in days). In this
paper, we present DeVRF, a novel representation to accelerate learning dynamic
radiance fields.....
This research is supported by National Research Foundation, Singapore and A*STAR, under its RIE2020 Industry Alignment Fund – Industry Collaboration Projects (IAF-ICP) grant call (Grant No. I2001E0059)
Jia-Wei Liu, Yan-Pei Cao, Weijia Mao, Wenqiao Zhang, David Junhao Zhang, Jussi Keppo, Ying Shan, Xiaohu Qie, Mike Zheng Shou
Problem Statement: A firm faces random demand for a service it delivers on a given future date. To boost demand, the firm hires a sales agent who exerts unobservable effort continuously over time. The firm is concerned not only with increasing current demand, but also with smoothing demand over time to avoid losing goodwill if realized demand exceeds available inventory. We model the firm’s incentive design problem using a continuous-time principal-agent framework, in which demand drifts over time in response to unobserved agent effort and the price the firm charges. To induce the agent’s sales effort, the firm chooses an incentive scheme that depends on the remaining inventory and the time to the service (e.g., time to departure in the case of airlines). We characterize the firm’s optimal incentive scheme .....
This research is supported by National Research Foundation, Singapore and A*STAR, under its RIE2020 Industry Alignment Fund – Industry Collaboration Projects (IAF-ICP) grant call (Grant No. I2001E0059)
SIA-NUS Digital Aviation Corporate Laboratory is a part of NUS’s Institute of Operation Research and Analytics.