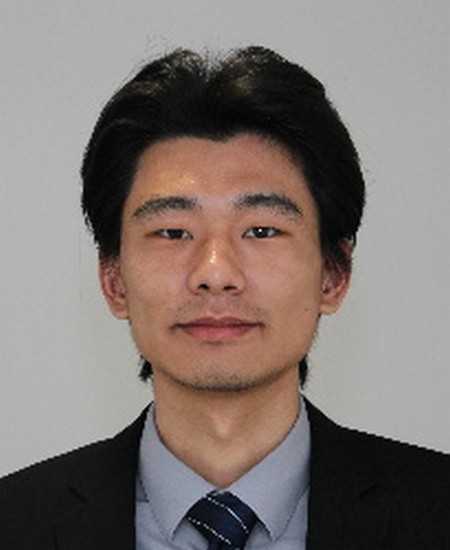
This work package aims to develop for SIA a new sense and response capability in the company to perform deep data analytics in airline revenue management under a changing environment.
Expertise, infrastructure, and relevant background work done at NUS’s Institute of Operations Research and Analytics (IORA) would be tapped on to add value to the proposed projects in this work package.
Where applicable, relevant projects in this work package will also be supported by Amadeus, a leading Pricing and Revenue Management Software provider and a partner of SIA, to provide additional access to industry-wide issues and datasets. Through the SIA-Amadeus partnership, SIA is also able to make accessible critical and real-time market data (owned by SIA) to the research team for the purpose of the proposed projects.
Price elasticity measures how the demand shifts with changes in prices. In order to estimate these quantities, the key is to understand the demand model as a function of prices and an assortment of choices. While this important issue has been addressed by various studies using various techniques, the approach used often assumes a simple demand-price structure and ignores the impact of seat allocation on the empirical booking dataset.
The team plans to use modern econometric and machine learning techniques to uncover the willingness-to-pay functions of the customers, in response to the assortment of fare classes offered to face the changing environment.
One of the central limitations in a standard revenue management system is the inability to learn and adapt to the environment. Many IT systems built for the revenue management teams are developed based on past observations and fixated views of the state of the world. Hence many pricing and revenue allocation decisions are made based on wrong assumptions.
In order to overcome these limitations, the team develops in this project a data-driven approach to understand the demand model faced in SIA. This is a key difficulty that has hindered research and development of data-driven approaches in the revenue management literature because of the difficulty to quantify or measure the potential of such approaches. More importantly, this project would allow SIA to validate and access the impact of such techniques on its RM performances.
In the airline revenue management system, the business and leisure passengers arrive at book tickets in different manners. For instance, business passengers normally book closer to the day of departure, whereas leisure passengers arrive more uniformly across the booking horizon. These assumptions are used to piece together the expected booking curve for passengers in different segments.
In order to mitigate the impact of the estimation errors in the expected booking curve, the team will analyse this problem in a setting where the demand is learned in a volatile environment.
The goal of this project is to derive a new seat allocation control algorithm, which can perform well without relying on the assumption of a specific booking curve. This study is inspired by recent advances in the use of online algorithms in the machine learning community.
There is a recent fundamental industry change in the airline sector: an increasing percentage of revenue now comes from ancillary items such as checked baggage, inflight food, premium seat selection, and extra-legroom, etc. Airlines have therefore an opportunity to adopt bundling tactics, product-suggestion analytics etc. to create customised recommendations for additional purchases, both at the original point of sale and over the course of the travel journey. Maximising total revenue depends on connecting a customer’s total spending data with that customer’s profile in the airline’s system. Doing this allows for attribute-level customisation, where airlines can segment customers into known groupings by location, demographics, and so forth.
In this project, the above semiparametric choice model will be used, where cross moments were used to model the correlation of preferences for a pair of products or services. In this way, we can convert the pricing problem into a semi-definite programming problem, where advanced solvers have been developed recently to solve this class of problems. We exploit the convexity result in this setting to develop an approximate solution to this difficult problem.
This project plans to develop a simulation engine for airline network revenue management, that can be used to replicate the booking arrival dynamic in many different situations and can be used as a digital twin to stress test any new pricing and seat allocation algorithms for the airline.
The team plans to develop a real-time simulation customised to SIA digital aviation ambition, and build in many other capabilities like consumer choice modelling beyond the traditional MNL models, and also sense and response system to provide early warning to change in the operating environment.
The team hopes to develop a unique platform for SIA, that can be further developed, customised, and used to stress test other creative ideas and parameters the aviation sector may have in the future. This project taps on and is dependent on the findings and results of the earlier projects in this work package, where the data collected, models derived and approaches arising from the projects would contribute towards the building of this simulation platform.
The successful development of this simulation platform will provide SIA with a key to “wargame” in real-time to visualise estimated impacts and market reactions to specified market actions or proposed strategic interventions by SIA.
Li Xiaobo, Sun Hailong and Teo Chung Piaw
Problem Statement: We study the bundle size pricing (BSP) problem where a monopolist sells bundles of products to customers, and the price of each bundle depends only on the size (number of items) of the bundle. Although this pricing mechanism is attractive in practice, finding optimal bundle prices is difficult since it involves characterizing distributions of the maximum partial sums of order statistics...
This research is supported by National Research Foundation, Singapore and A*STAR, under its RIE2020 Industry Alignment Fund – Industry Collaboration Projects (IAF-ICP) grant call (Grant No. I2001E0059)
Yan Zhenzhen, Teo Chung Piaw, Karthik Natarajan, Cong Cheng
Problem Statement: We develop a data-driven approach for the multi-product pricing problem, using the theory of a representative consumer in discrete choice. We establish a set of mathematical relationships between product prices and demand for each product in the system, including that of the outside option. We provide identification conditions to recover the underlying representative consumer model and show that with sufficient pricing experiments, the approach can identify the underlying demand model (more precisely, the associated perturbation function in the representative consumer model) accurately, up to a constant shift and a given tolerance level...
This research is supported by National Research Foundation, Singapore and A*STAR, under its RIE2020 Industry Alignment Fund – Industry Collaboration Projects (IAF-ICP) grant call (Grant No. I2001E0059)
Maoqi Liu, Li Zheng, Changchun Liu, and Zhi-hai Zhang.
Problem Statement: We study the product design problem where a decision maker selects the features of a product from a set of feasible options. We focus on two widely studied objectives in this field, that is, the share of choice (SOC) and buyers' welfare (BW). The two objectives are vulnerable to different types of customer preference misspecification, that is, deviation from the nominal utility distribution and effects of outliers, respectively....
This research is supported by National Research Foundation, Singapore and A*STAR, under its RIE2020 Industry Alignment Fund – Industry Collaboration Projects (IAF-ICP) grant call (Grant No. I2001E0059)
Ju Liu, Changchun Liu, Chung Piaw Teo
Problem Statement: We develop a general framework for selecting a small pool of candidate solutions to maximize the chances that one will be optimal for a combinatorial optimization problem, under a linear and additive random payoff function. We formulate this problem using a two-stage distributionally robust model, with a mixed 0–1 semidefinite program. This approach allows us to exploit the “diversification” effect inherent in the problem to address how different candidate solutions can be selected to improve the chances that one will attain a high ex....
This research is supported by National Research Foundation, Singapore and A*STAR, under its RIE2020 Industry Alignment Fund – Industry Collaboration Projects (IAF-ICP) grant call (Grant No. I2001E0059)
SIA-NUS Digital Aviation Corporate Laboratory is a part of NUS’s Institute of Operation Research and Analytics.